Transforming Data Annotation: The Key to Unlocking AI Potential
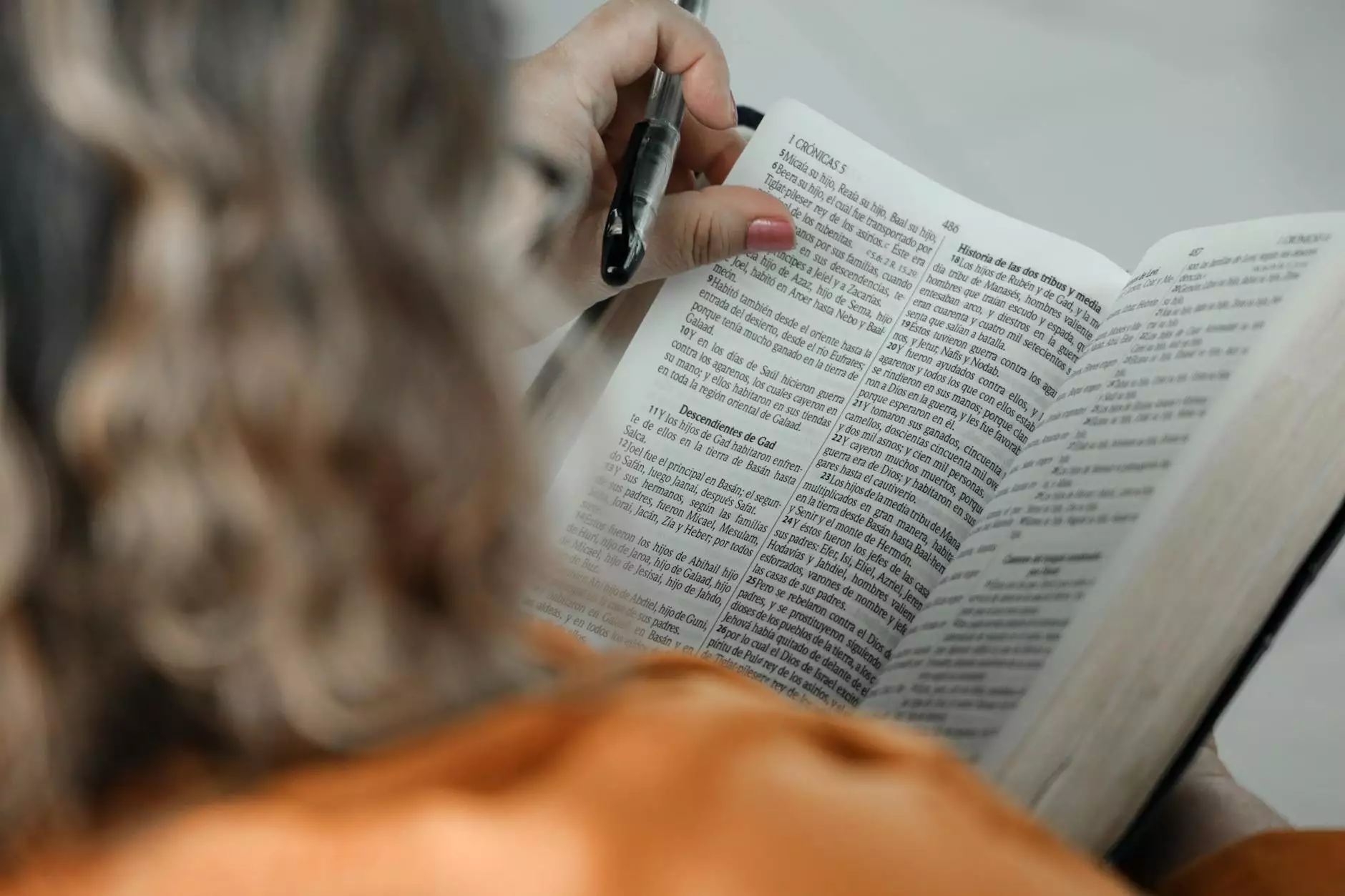
Data annotation has become a crucial aspect of the increasingly digital world we live in. As businesses strive to harness the power of artificial intelligence (AI), the importance of quality data annotation cannot be overstated. In this article, we delve into the significance of data annotation tools and platforms, and how they can propel businesses forward. For more insights and advanced solutions, visit https://keylabs.ai/.
The Essence of Data Annotation in AI Development
At the heart of AI and machine learning lies the need for high-quality, organized data. Data annotation is simply the process of labeling or tagging data to help machines understand it. This is fundamental for training AI models, which rely on annotated datasets to learn and make predictions.
Why Quality Matters in Data Annotation
When it comes to data annotation, quality is paramount. Poorly annotated data can lead to inaccurate models, mispredictions, and ultimately, a loss of resources. Here are some critical aspects that highlight the importance of quality in data annotation:
- Accuracy: High-quality annotations ensure that the AI model learns correctly, enhancing its performance.
- Consistency: Consistent annotations across datasets reduce confusion and improve model reliability.
- Scalability: Efficient annotation processes allow businesses to scale their data efforts as needed.
- Cost-effectiveness: Investing in quality data annotation reduces long-term costs by preventing model errors and reducing the need for rework.
Types of Data Annotation Tools
Data annotation tools vary in functionality and features, tailored to meet the diverse needs of different industries. Here’s a look at some popular types of data annotation tools available today:
1. Image Annotation Tools
These tools label images for computer vision applications. Annotations may include:
- Bounding boxes: to identify objects within images.
- Semantic segmentation: to delineate boundaries between different objects.
- Keypoint annotation: for tasks like facial recognition.
2. Video Annotation Tools
Video annotation is vital for various applications, especially in security and traffic analysis. Common tasks include:
- Object tracking: to monitor the movement of items or people.
- Scene classification: categorizing video content.
- Action recognition: identifying activities within the video.
3. Text Annotation Tools
Text annotation is essential for natural language processing (NLP) tasks. Some examples include:
- Sentiment analysis: labeling text to determine emotional tone.
- Entity recognition: identifying names, dates, and places.
- Text classification: sorting texts into predefined categories.
Exploring Data Annotation Platforms
A data annotation platform integrates various tools and resources to facilitate efficient data labeling processes. The ideal platform will offer:
- User-friendly interfaces: Simplifying the annotation process for annotators.
- Collaboration features: Enabling teams to work together seamlessly.
- Quality assurance mechanisms: Ensuring the accuracy and reliability of annotations.
- Scalability options: Supporting large datasets and multi-project management.
Key Benefits of Utilizing Data Annotation Tools and Platforms
Organizations that leverage data annotation tools and platforms can expect numerous benefits, including:
1. Enhanced Data Quality
Automated tools help maintain high data quality through consistent annotations and validation checks.
2. Increased Efficiency
With user-friendly platforms, businesses can streamline their data annotation processes, significantly reducing time-to-market for AI solutions.
3. Cost Reduction
Companies can cut costs by reducing the need for manual labeling and rework, allowing teams to allocate resources more effectively.
4. Improved Collaboration
Integrated platforms promote collaboration among stakeholders, enhancing communication and project management.
5. Greater Accuracy
High-quality annotation tools help ensure that AI models receive accurate data, increasing the chances of successful deployment.
How to Choose the Right Data Annotation Tool or Platform
Selecting the right data annotation tool or platform involves several considerations. Here are some factors to keep in mind:
- Project Requirements: Assess the specific data types your project involves, such as images, videos, or text.
- User Experience: Choose a platform that is intuitive and easy for your team to use without extensive training.
- Integration Capability: Ensure that the tool integrates well with your existing systems and workflows.
- Cost Structure: Analyze pricing models to find a solution that fits your budget and delivers value.
- Support and Training: Opt for platforms offering robust customer support and training resources to help your team succeed.
Real-World Applications of Data Annotation
Data annotation finds applications across numerous industries. Here are some notable sectors benefiting from advanced annotation tools and platforms:
1. Healthcare
In healthcare, annotated medical images assist in diagnosing diseases and enhancing patient outcomes through precise AI insights.
2. Autonomous Vehicles
Self-driving cars rely on image and video annotations to recognize road signs, obstacles, and pedestrians, ensuring safety on the roads.
3. Retail and E-commerce
Retail companies use data annotation to analyze customer sentiment and enhance user experience by personalizing offerings.
4. Finance
In the financial sector, annotated data aids in fraud detection and risk assessment, leading to more secure and efficient operations.
5. Agriculture
Precision agriculture leverages image annotations for crop monitoring and yield prediction, optimizing farming practices and resources.
The Future of Data Annotation Technologies
As AI technologies evolve, so do data annotation tools and platforms. We can expect advancements in areas such as:
- Automation: Enhanced automation will streamline workflows and reduce manual intervention further.
- AI-Assisted Annotation: AI is being integrated into tools to speed up the annotation process while improving accuracy.
- Real-time Collaboration: Future platforms will likely offer more robust real-time collaboration features, essential for global teams.
- Expanded Data Types: Tools will accommodate diverse data types, including audio and complex 3D environments.
Conclusion: Empowering AI with Robust Data Annotation Solutions
In conclusion, the role of data annotation tools and platforms in the development of AI cannot be underestimated. They are vital in ensuring that the data feeding into AI systems is of the highest quality, driving innovation and success across various industries. Organizations that invest in these solutions, like those offered at https://keylabs.ai/, position themselves at the forefront of technology, enhancing their AI capabilities and achieving their strategic goals.
Explore the transformative potential of data annotation today, and unlock the true capabilities of artificial intelligence for your business! Invest in the right tools, harness quality annotation practices, and ensure your AI models deliver accurate and reliable outcomes.