Revolutionizing Cybersecurity with Machine Learning Malware Detection
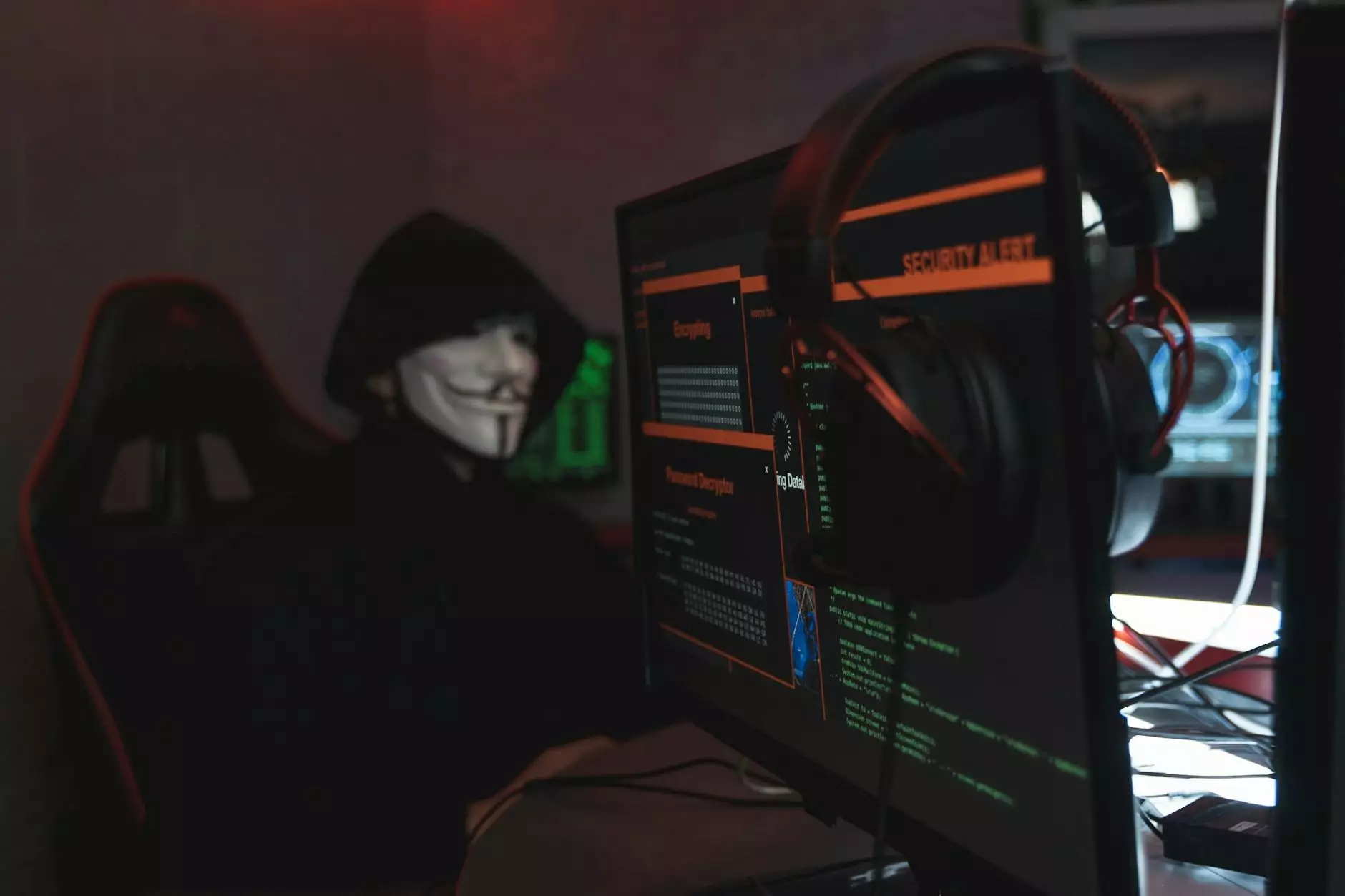
In the digital landscape of the 21st century, businesses face unprecedented challenges regarding cybersecurity. As cyber threats become more sophisticated, traditional security measures often fall short. Fortunately, advancements in technology, particularly in machine learning malware detection, are paving the way for more robust and effective security solutions. In this article, we will delve deep into how machine learning is revolutionizing the way we detect and respond to malware, ultimately safeguarding the integrity of our businesses.
Understanding the Basics of Machine Learning and Malware Detection
Machine learning is a subset of artificial intelligence that enables systems to learn from data and improve their accuracy over time without being explicitly programmed. In the realm of cybersecurity, machine learning can analyze vast amounts of data to identify patterns and behaviors associated with potential threats.
On the other hand, malware detection refers to the process of identifying malicious software designed to harm or exploit any programmable device or network. Combined, these fields work in harmony to create a formidable defense against the evolving landscape of cyber threats.
The Importance of Machine Learning in Cybersecurity
As reported by cybersecurity experts, the number of cyber attacks has increased dramatically over the past years. Small and medium-sized enterprises (SMEs) are particularly vulnerable, lacking the resources to implement comprehensive security measures. This is where machine learning malware detection comes into play:
- Increased Accuracy: Unlike traditional methods that often rely on signature-based detection, machine learning algorithms can recognize and adapt to new threats by analyzing behavioral patterns.
- Real-time Threat Assessment: With instant analysis capabilities, organizations can swiftly respond to emerging threats before they can cause substantial damage.
- Reduced False Positives: Machine learning models continuously learn and evolve, leading to a decrease in false alarms compared to conventional methods.
- Scalability: As businesses grow, the amount of data that needs monitoring grows too. Machine learning systems can scale easily to handle larger datasets.
How Machine Learning Malware Detection Works
Machine learning malware detection operates through several methodologies, primarily focusing on analyzing historical data and identifying anomalies:
1. Data Collection
The first step involves the collection of data from various sources such as files, network traffic, and system behaviors. This historical data is critical for training machine learning models.
2. Feature Extraction
Once data is collected, the next step is to extract significant features that may indicate malicious behavior. This could include file size, file type, API calls, and other relevant attributes.
3. Training Models
Using the extracted features, machine learning models are trained. During this phase, algorithms learn to distinguish between benign and malicious software based on previously identified patterns.
4. Real-time Detection
After training, the models are deployed in a production environment. They continuously analyze incoming data against the learned patterns to detect any anomalies that may signify a malware attack.
Types of Machine Learning Algorithms Used in Malware Detection
There are several machine learning algorithms commonly used in the field of malware detection:
- Decision Trees: These algorithms model decisions as branching paths, making it easy to visualize and understand the decision-making process.
- Random Forests: An ensemble of decision trees that improves the accuracy of predictions by considering multiple trees rather than a single model.
- Support Vector Machines (SVM): SVMs are effective in high-dimensional spaces and are used to classify data into different categories.
- Neural Networks: These are particularly powerful for detecting complex malware signatures and patterns through deep learning techniques.
The Challenges of Implementing Machine Learning in Malware Detection
Despite its advantages, integrating machine learning malware detection into cybersecurity frameworks does come with its challenges:
1. Data Quality and Quantity
The success of machine learning models highly depends on the quality and diversity of data. Inadequate or biased data can lead to ineffective models.
2. Model Interpretation
Many machine learning algorithms, especially deep learning models, operate as "black boxes," making it hard to interpret how decisions are made. This lack of transparency can hinder trust in automated systems.
3. Evasion Techniques
Cybercriminals are constantly developing new methods to evade detection by understanding how machine learning models work. They exploit vulnerabilities to create zero-day attacks.
Case Studies: Success Stories of Machine Learning in Malware Detection
Many organizations have successfully implemented machine learning malware detection, leading to significant improvements in their cybersecurity posture. Here are a few examples:
1. Company A: Fortune 500 Manufacturer
Company A, a leading manufacturer, faced numerous cybersecurity incidents due to outdated malware detection systems. By integrating machine learning technologies, they reduced their incident response time by over 75%, significantly improving their overall security.
2. Company B: Financial Sector Institution
A financial institution adopted machine learning algorithms to protect sensitive customer data. The implementation resulted in a 30% decrease in confirmed security breaches and transformed the organization’s approach to cybersecurity, ensuring compliance with financial regulations.
Best Practices for Leveraging Machine Learning Malware Detection
To maximize the benefits of machine learning in malware detection, organizations should consider the following best practices:
- Invest in Quality Data: Ensure that the data used for training models is comprehensive, diverse, and of high quality.
- Regularly Update Models: Cyber threats evolve rapidly, so continuously updating machine learning models is crucial for maintaining their effectiveness.
- Enhance Collaboration: Foster communication between data scientists and cybersecurity teams to tailor machine learning models effectively.
- Implement Multi-layered Security: Rely on machine learning as part of a broader cybersecurity strategy that includes firewalls, intrusion detection systems, and employee training.
The Future of Machine Learning in Malware Detection
The potential for machine learning malware detection remains vast. As technology continues to evolve, we can expect significant advancements in capabilities:
- Artificial General Intelligence: Future machine learning systems may reach higher levels of adaptability and decision-making capabilities.
- Integration with Blockchain: Implementing blockchain technology could enhance data integrity and further secure the machine learning processes.
- Collaborative Defense Systems: Organizations may work together to share data on emerging threats, improving models collectively.
Conclusion
In conclusion, machine learning malware detection represents one of the most significant advancements in cybersecurity. By leveraging its capabilities, organizations can protect themselves from an evolving array of threats, ensuring business continuity and safeguarding sensitive data. The integration of machine learning not only enhances detection accuracy but also streamlines response protocols, making it a vital component of modern cybersecurity strategies. As cyber threats become more complex, the adoption of machine learning will be paramount for organizations aiming to stay ahead in the arms race against cybercriminals.
For businesses looking to implement robust cybersecurity solutions, exploring machine learning capabilities is a step toward a more secure future. At Spambrella, we offer comprehensive IT services & computer repair as well as state-of-the-art security systems that can help your organization thrive in a safe digital environment.